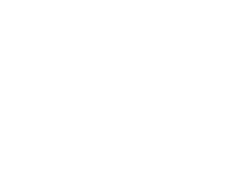
Element Four: Iterative and Experimental Process
• Emphasis on generating a portfolio of possible solutions, rather than a single “optimal” one
• Treating these potential solutions as hypotheses to be tested and adapted through iteration and feedback from actual users
• Creation of low-fidelity prototypes (less refined than The Lean Startup’s minimum viable product) to support this experimentation and then co-creating towards higher-fidelity prototypes with stakeholder feedback
In traditional decision-making approaches, managers are taught to believe in the ability to analyze and optimize—to select the one “best” solution. This approach works under conditions of stability when past data accurately predicts the future, rarely the case when the goal is innovation and a new future is sought. Contrast traditional decisionmaking steps with innovation:
• Identify the problem—yet it is often conventional framing and obvious problem definitions that reduce the ability to see more creative alternatives.
• Lay out and evaluate the options—yet in most cases where innovation is sought, the easily identified options are not attractive. Nor in an uncertain environment is good data available to evaluate them.
• Finally, choose the optimal one—but how can optimality be defined when key stakeholders lack shared criteria, have differing definitions of the issue and there is little data relating cause-to-effect?
Luigi Ferrara, dean of the Centre for Arts, Design and Information Technology at Toronto’s Institute without Boundaries, underlines the impact of design thinking’s focus on action and its emphasis on prototyping and experimentation. According to Ferrara, when you must produce something concrete, thinking becomes focused. Ferrara notes that staying safely in the debate space is comfortable and easy because one’s hypothesis never interacts with reality to get feedback whether or not it is true. “It’s what paralyzes bureaucracies,” he says. “You can debate forever. This is where design gets interesting. A fundamental part of design is making things sharable in the world. That forces collaboration because you have to agree on an output. And that changes the thinking. You can say, ‘We want to be the world’s best city,’ but that is really empty until you confront the design challenge: ‘What is the best city?’ All of a sudden a bunch of qualities come out, and those qualities need to be shared.”
Like venture capitalists who invest in 10 start-ups expecting less than two to succeed, design thinkers distrust their ability to predict winning approaches and believe that multiple, but smaller, bets are essential. A design thinking goal, in uncertain contexts, is to learn through rapid experiments. Learning in action as quickly and cheaply as possible rather than a priori analysis allows multiple iterations of prototyping, feedback, and refining so that teams can detect failure before an organization has made an expensive bet on any given idea.
For decades, cognitive scientists have explored flaws in decision makers’ hypothesis-testing abilities, including over-optimism, inability to see disconfirming data, attachment to early solutions, and a preference for the easily imagined.12 Training in the design and execution of experiments reduces these cognitive biases. Design offers structured tools for surfacing and testing assumptions that bring to the fore unexamined suppositions and identify the data needed to test them.
We find that analytically-oriented managers, generally taught to turn immediately to spreadsheets and already-existing data to test ideas, often struggle with hypothesis-driven thinking. In design, innovators identify what data or stories are needed to analyze the particular assumption in question, and then develop a strategy for obtaining it. This reversal, though easy to discuss in theory, is challenging in practice for managers schooled in traditional problem solving.
Experimental approaches impact innovation outcomes by minimizing both the investment in and the visibility of failures, especially important in bureaucratic, risk-averse environments, and mitigate well-recognized decision biases that interfere with effective problem solving. Especially important where employees fear failure and are stymied by slow decision processes, design thinking encourages a learning mindset and action orientation.
Though it seems counter-intuitive, human-centered decision processes—in spite of the upfront emphasis on exploration and dialogue—regularly take less time than traditional organizational rollouts because iterative experiments also are under-the-radar communications which pave the way for project acceptance by those who must implement them.