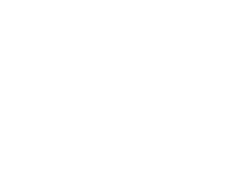
AI Literacy: A Prerequisite for the Future of AI and Automation in Government
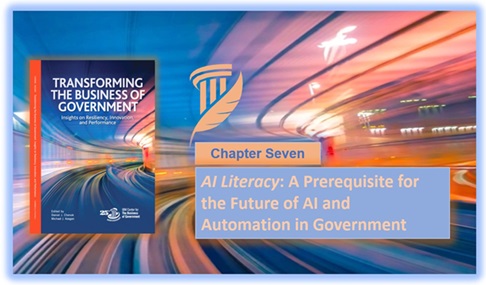
We turn from resiliency to the topic of innovation. The next nine chapters will address larger themes of innovation and performance to assist government leaders in efforts to enhance agency readiness.
With the rapid progression of artificial intelligence and automation technologies, the public sector is in a state of transformation. Government leaders and managers are on the frontlines, responsible for harnessing AI’s potential into tangible results. Numerous public efforts have been made to address AI’s design, deployment, and maintenance, such as establishing the National Artificial Intelligence Initiative1 and the Blueprint for an AI Bill of Rights2 developed by the White House Office of Science and Technology Policy (OSTP). These pragmatic advancements aim to guide AI use across agencies and create a system for documenting use cases and principles. But one key assumption often underpins these developments—that stakeholders already have a comprehensive understanding of what AI is and how it can be leveraged across their workflows.
A new focus has emerged: cultivating strategy to enhance AI literacy. Originating from computer science, information studies, and learning sciences, AI literacy involves understanding the technical facets of AI and learning how to leverage it in practice. In a study from the Georgia Institute of Technology, researchers define general AI literacy as “a set of competencies that enable individuals to evaluate AI technologies critically; communicate and collaborate with AI; and use AI as a tool.”3 The push for more explainable, responsible, trustworthy, and transparent AI has been part of this shift. AI literacy requires not just learning but learning to learn—asking the right questions to comprehend how AI systems work. This requires understanding a tool’s capabilities, its limits, ethical implications, and how to incorporate it into operations.
Blueprint for Building AI Literacy
This chapter delves into the vital role of strategic actions for AI literacy, particularly for leaders and managers navigating the intricacies of an increasingly auto- mated workplace. It outlines a three-phased approach outlined in Figure 1. for boosting AI literacy, presenting key actions and practices to make government organizations more responsive and fundamentally reshaping them to deliver exceptional public services and achieve mission success. By adopting these strategic actions for AI literacy, governments can ensure that ongoing advancements in AI and automation are harnessed effectively and ethically, providing the greatest possible benefit to the public. This approach offers a crucial tool for government leaders and managers, helping them navigate the complexities of AI implementation within their organizations.
Assessment Phase
Develop AI vision and goals. The initial step in enhancing AI literacy within an organization involves establishing clear, actionable goals. These objectives should be tailored to the unique needs of each organization, reflecting their specific context and use cases for AI. For instance, one organization might focus on understanding how AI can augment efficiency in routine tasks, while another might concentrate on understanding the ethical implications of AI in handling sensitive data.
Leaders are responsible for ensuring these goals align with the organization’s mission, values, and strategic objectives. For instance, if a pillar of an organization’s mission is to enhance customer service, an AI literacy goal could involve understanding how automation can be deployed for improved client interaction and engagement. When establishing AI goals, organizations should also consider potential challenges associated with AI integration, such as data privacy, ethical use, and technical capacity. For example, a team handling sensitive data might prioritize learning and mitigating privacy risks associated with an AI tool. Similarly, other teams with limited technical expertise might focus on building fundamental conceptual knowledge before using more advanced technology applications. These goals should be flexible and evolve alongside technological change and organizational growth.
The term ‘AI’ covers a broad spectrum including algorithms, machine learning models, generative AI, recommender systems, neural networks, robotics, design principles, and industry trends. Acknowledging this diversity is crucial as leaders define AI’s role within their organization and set corresponding literacy goals. The spectrum of learning should also be considered in the goal-setting process. Achieving AI literacy is a progressive journey, and goals can span a continuum, from understanding basic AI concepts and applications to comprehending more complex aspects—such as deciding the amount of risk arising from automating high-reliability decisions.
Given the emergence and widespread adoption of new forms of AI like generative AI, encompassing technologies like ChatGPT, Bard, and GitHub Copilot, leaders can consider how to define and set AI literacy goals. This shift involves more than just understanding AI’s mechanics and using it responsibly. For instance, generative AI fosters dynamic user interaction, paving the way for creating new content and solutions to complex problems. Notably, this technological advancement is democratizing AI usage, transforming users from being primarily consumers of AI outputs to active creators with AI. In this context of rapidly evolving technology, the value of developing robust, flexible frameworks for AI literacy is key. Even as technologies change, the processes to comprehend, use, and enhance them remain crucial. Therefore, AI literacy goals must be conceptualized as dynamic and adaptive, ready to accommodate these evolving forms of inter- action with technology. This will ensure that the workforce is well-prepared for the future.
Assess current AI literacy levels. With goals outlined, the subsequent step is to assess the current state of AI literacy across different levels in an organization. This assessment forms an essential baseline, shedding light on existing AI understanding within leadership and illuminating areas requiring enhancement. AI literacy benchmarks play a vital role here, providing a well-defined standard against which the organization’s AI knowledge can be evaluated. These benchmarks serve as a roadmap, guiding the organization to identify gaps, set realistic improvement targets, and track progress over time.
A significant part of AI literacy is developing data literacy as a competency. Data literacy refers to reading, understanding, creating, and communicating data as information. In the context of AI, this means understanding how AI systems collect, process, and interpret data. It also involves maintaining relationships with vendors to ask the right questions and unravel the complexities of these processes to ensure data literacy principles become embedded into tools.
However, the knowledge of AI and data should not be the endpoint. Leaders should decide where AI fits within the organization’s operational framework. This can be achieved by conducting an audit of the existing workflow, identifying tasks that could be automated, processes that might face disruption and opportunities for new AI-enabled processes. If feasible, conducting internal surveys, interviews, or focus groups can offer invaluable insights during this assessment phase. The information gathered outlines the current state of AI literacy and aids in creating tailored educational programs. These programs should address the organization’s leaders and managers’ specific learning needs and knowledge gaps.
In addition to the technical aspects of AI, a human factors perspective plays an integral role in successful AI adoption. AI literacy assessments should evaluate employees’ attitudes toward automation technologies. Understanding these sentiments can highlight potential barriers to AI acceptance and indicate any areas of resistance stemming from the organization’s culture. The insights from this evaluation could help in developing communication adoption strategies and training programs that address these apprehensions. Further, it could help identify potential ‘intelligence officers’ or ‘translators’ within the organization. These specialists understand a tool’s technical details and its potential application across various organizational departments. They serve as a bridge between AI specialists and operational staff to communicate how technology initiatives can contribute to larger organizational practices and goals. By integrating these human factors into AI lit- eracy assessments, leaders can foster an environment that supports a more complete, inclusive transition toward AI adoption.
Implementation Phase
Adopt a co-creation approach for AI implementation. A co-creation strategy in AI implementation is indispensable for effective technology integration within organizations. This process is characterized by a back-and-forth dialogue between developers and end-users of AI technologies. A case study led by Stanford University in the healthcare sector provides an example.4 Two machine learning tools—predicting potential bed shortages and estimating patient readmission rates—were developed through an iterative co-creation process between developers and employees in a health system.
In the context of government agencies, the co-creation process might include several elements. First, developers and operational managers collaboratively identify potential AI solutions that align with the agency’s needs and technical capabilities. Then, these proposed solutions are refined through continuous engagement with a broader group of stakeholders, including other department leaders or external experts. A prototype of the AI tool is then implemented on a pilot basis, allowing end-users—in this case, managers and staff—to provide real-time feedback and identify potential discrepancies or areas of improvement. The final stage involves refining the tool based on user feedback, adjusting the AI models, and ensuring the tool is both user-friendly and effective.
By fostering and practicing collaborative design, this approach can enable employees and leaders with a shared responsibility for the development of a tool, which is created collectively through practice and aims to address an employee’s workflow. This co-creation process can be an opportunity for building trust and task-technology ownership among staff. As employees become involved in the development and refinement of AI tools, they can gain a deeper understanding and appreciation of the technology. This can help alleviate apprehension and resistance, promoting acceptance and successful integration of AI across operations.
Promote interagency agility. Building a future with successful AI integration necessitates collaboration and awareness, not just within a single organization but across a broad network of agencies. As leaders engaging with and leveraging these inter- agency connections is critical.
One key practice involves sharing each organization’s AI use cases, challenges, and solutions with other agencies. The National Artificial Intelligence Initiative encourages this dialogue by requiring federal agencies to annually report an inventory of how they use AI in tasks and to share the database with other government agencies and the public.5 This becomes increasingly relevant as a survey by Accenture reported that 76 percent of leaders across 16 industries struggle with understanding how to maximize AI value across operations.6 Such a knowledge exchange serves as a platform for learning from others’ experiences, facilitating the adoption of proven strategies, and unveiling potential uses of AI.
Leaders can both contribute to and benefit from a shared repository of knowledge and experience. This approach relies on a process of social influence, a concept rooted in social psychology, where peers’ shared experiences and successes can positively impact the likelihood of wide- spread technology adoption. By championing inter-agency collaboration and fostering an internal culture of AI awareness, both an organization and the broader government network can be better positioned for successful technology adoption.
Ensure responsible and trustworthy AI use. The responsible usage of AI, characterized by elements that include fairness, transparency, privacy, and explainability, is paramount when integrating this technology into government operations. Yet, responsible use is only one side of the coin. Trustworthiness is another critical aspect, referring to the dependability of AI in producing accurate, consistent outputs under a wide range of circumstances. Ethical guidelines for AI use must be incorporated into organizational policies and procedures, enabling leaders to make informed and ethical AI-related decisions. For example, the Department of Defense’s five principles of AI ethics—responsibility, equity, traceability, reliability, and governability—provide a strong foundation. This involves a thorough understanding of the technology, rigorous testing of AI capabilities, and implementation of systems to detect and mitigate unintended consequences.
Trustworthy AI requires systems to be versatile and reliable, functioning correctly under various situations and consistently delivering on their intended functions. This entails rigorous testing and quality assurance processes that scrutinize the system’s performance. Trustworthy AI also emphasizes the reproducibility and verifiability of results. Auditability is a critical facet to achieving these principles. It involves the ability to develop processes to inspect and review the workflows the AI model employs to make decisions. This enables accountability and transparency in AI operations. Moreover, a significant aspect of trustworthy AI includes providing redress mechanisms. If the AI system makes an error, it is crucial to have procedures in place for affected parties to seek remedy or correction.
A principle that binds responsible and trustworthy AI together is user over- sight. While delegating decisions to AI systems can increase efficiency, users must retain control and the ability to intervene when necessary. This serves as a safeguard, ensuring that technology serves human-centric values and ethical norms. Several collaborative initiatives are shaping this area, under- lining the importance of broad stakeholder input. For instance, the National Telecommunications and Information Administration (NTIA) actively seeks feedback from various agencies to inform policies that support the development of AI audits, assessments, and certifications, thereby promoting trust in AI systems.7 Similarly, the National Institute of Standards and Technology (NIST) has established the Trustworthy and Responsible AI Resource Center as a platform to foster trustworthiness in designing, developing, using, and evaluating AI products, services, and systems.8 At both the state and federal levels, these collective efforts provide leaders with resources for developing ethical AI guidance.
AI systems can reflect and amplify societal biases and potentially lead to unfair outcomes. Moreover, the vast quantities of data collected and processed by AI systems pose a significant target for cyberattacks, data breaches, and misuse. Ensuring responsible AI use is an ongoing, complex, yet crucial task. It requires collaboration across all organizational levels and roles—whether from the intelligence officer who anticipates potential issues, the technical specialists who troubleshoot problems, or the operations personnel who implement the solutions. Each person, no matter their role, must have some form of AI literacy. This unified approach underscores the importance of everyone having a stake in understanding and managing AI technologies.
As leaders, being aware of these challenges and using existing frameworks, or developing new ones as necessary, is an essential part of this task. In addition to implementing ethical guidelines, organizations should also establish mechanisms for monitoring and reviewing the use of AI. This involves regular auditing and evaluations to ensure that AI systems are operating as intended and ethical standards are being upheld. This broad and cooperative approach ensures a thorough, organization-wide commitment to responsible and trustworthy AI use.
Evaluation and Continuous Learning Phase
Measure progress and adjust. Developing a generalized blueprint for AI literacy poses a unique challenge given the diversity of agencies, each with its distinct needs, resources, and regulations. However, a critical and shared step involves regularly assessing progress toward AI literacy goals and subsequent adjustments. Regular tracking can be achieved through follow-up assessments, feedback sessions, or performance reviews. Progress measurement should also consider qualitative aspects, such as employees’ confidence and comfort in using AI tools, their understanding of AI’s capabilities and limitations, and their efficacy in making informed decisions with their tools. These measures not only provide insight into the learning curve but also identify areas for improvement or adjustment in strategy. Achieving AI literacy is not a static goal but a dynamic, ongoing process. The feedback collected during this stage is invaluable for refining strategies, and ensuring that learning initiatives remain effective, relevant, and aligned with the organization’s AI literacy goals.
Provide regular training and foster learning. Maintaining AI literacy necessitates fostering an environment of continuous learning. Leaders should ensure regular training sessions through various partnerships with universities, industry experts, and specialized in-house training initiatives. These partnerships provide leaders and managers with access to the latest AI developments and trends.
Creating an AI-literate culture is a strategic move that contributes significantly to the acceptance and effective use of AI technologies. This can involve organizing awareness campaigns, seminars, and workshops that not only demystify AI’s black box, but also illuminate the potential of AI in enhancing public service delivery. Leadership plays a pivotal role in embedding this culture within the organization. By educating their teams about AI’s capabilities and implications through various learning initiatives, they ensure to leverage AI strategically.
Looking Forward: Advancing AI Literacy
The strategic actions outlined in this chapter form a comprehensive approach to enhancing AI literacy within government operations. These actions—including setting clear goals, assessing current AI understanding, implementing technology through a co-creative approach, promoting inter-agency awareness, ensuring responsible use, and fostering a culture of continuous learning—are all key elements in this transformative process. Building a future where AI and automation enhance government operations and resonate with an AI-literate workforce requires a concerted effort from all stakeholders. The emphasis on AI literacy signifies a shift in mindset towards viewing emerging technologies not as mere tools, but as a strategic partner in enhancing public service delivery.
Ultimately, this three-phased approach aims to equip government leaders and managers with the insights and actions necessary to navigate the complexities of AI integration. Furthermore, these strategies can empower leaders to discern the potential of new tools confidently, paving the way for informed decisions about adopting and integrating cutting-edge technologies. By fostering an environment where AI literacy is at the forefront of organizational strategy, government agencies can become more efficient, responsive, and ultimately, advance their mission for the citizens they serve.
Ignacio F. Cruz is an Assistant Professor of Communication at Northwestern University. His research focuses on the Future of Work, specifically how organizations strategically design, implement, and assess emerging technologies in their workflows.
Endnotes
1 National Artificial Intelligence Initiative Office, “Legislation and Executive Orders,” 2023, https://www.ai.gov/legislation-and-executive-orders/.
2 White House Office of Science and Technology Policy (OSTP), “Blueprint for an AI Bill of Rights: Making Automated Systems Work for the American People,” 2022, https://www.whitehouse.gov/ostp/ai-bill-of-rights/.
3 Long, Duri and Brian Magerko, “What is AI Literacy? Competencies and Design Considerations,” in Proceedings of the 2020 CHI Conference on Human Factors in Computing Systems (Association for Computing Machinery, 2020), 1-16, https://dl.acm.org/doi/10.1145/3313831.3376727.
4 Singer, Sara J., et al., “Enhancing the Value to Users of Machine Learning-Based Clinical Decision Support Tools: A Framework for Iterative, Collaborative Development and Implementation,” Health Care Management Review 47, no. 2 (2022): E21-E31, https://journals.lww.com/hcmrjournal/Abstract/2022/04000/Enhancing%20_%20the%20_%20value%20_%20to%20_%20users%20_%20of%20_%20machine.11.aspx
5 National Artificial Intelligence Initiative Office, “AI Use Case Inventories,” 2023, https://ai.gov/ai-use-cases/
6 Accenture, AI: Built to Scale Report, 2019, https://www.accenture.com/content/dam/accenture/final/a-com-migration/thought-leadership-assets/accenture-built-to-scale-pdf-report.pdf
7 National Telecommunications and Information Administration, “AI Accountability Policy Received Comments,” 2023.
8 National Institute of Standards and Technology, “Trustworthy & Responsible AI Resource Center,” 2023, https://airc.nist.gov/Home.