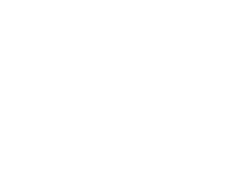
The Future of AI For the Public Sector: The Challenges and Solutions
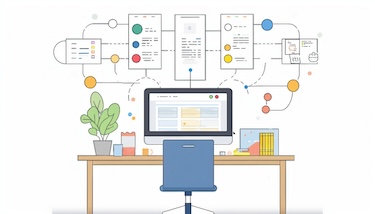
How best to take advantage of the promise of AI while mitigating risks was a primary topic at a gathering of 30 AI experts last year. The first installment of a four-part series drawn from this conversation focused on the benefits of AI. Our second blog began the conversation of the roundtable’s views on key challenges and solutions that need to be addressed to achieve such benefits.
This third blog post addresses the challenges and potential solutions regarding data and bias. We begin with four challenges:
- AI can pick up opinions and understand them as fact, which are then used to make decisions. But the confusion between opinions and fact is a significant one, and when they are conflated, public sector leaders can be misled.
- When a government yields the interpretation of data to artificial intelligence, it may inappropriately give up the power to make decisions based on instinct and human experience. All too often the algorithms that form the backbone of AI systems have been developed by technology experts without sufficient consultation with human beings on the front lines who really understand the way things work. For example, said one participant, “Social workers have not been in the loop when (using) AI in the agencies.”
- The most hazardous issue that came up repeatedly in the conversation was the potential of bias. There was no suggestion made in the conversation that governments are purposefully building bias into the algorithms, but unintended biases can easily creep in. However, as one participant explained, “AI requires that we develop algorithms, and if those algorithms have implicit bias built into them, it's going to exacerbate, not improve, the situation.”
- Ultimately, the problems with bias in AI become particularly acute when “the decision-making process is influenced inappropriately by the use of AI,” said another member of the roundtable. And when bias creeps into the systems the burgeoning use of AI can lead to harm. As another participant pointed out, “Trust in institutions is dwindling,” and when biases are uncovered, that phenomenon will only be exacerbated.
Solutions addressing bias and the need for human interaction include:
- Participants suggested that governments must hold themselves – and their contractors accountable to avert this undesirable outcome. “So, for example,” said one participant, if an entity was “applying for a grant in child welfare, you’d want to include an evaluation of the implicit bias” in that system.
- A potential way to avoid accusations of bias, said one participant, is to make sure that you have “people who are reflective of society overseeing the development of those models.”
- It’s also important to keep human beings in the equation, by involving the people who are going to utilize these new tools in their development and implementation. It’s about “having somebody who's a content expert in addition to an obviously technical expert.”
- “We need humans in the loop,” said one participant who added that it was important to identify who is the responsible party for AI in an entity. There should be “coordination over expertise and (the ability to) compare use cases . . . in AI procurement.”
- Unsurprisingly, as one participant noted, an important element for this work is “training, training, training, all over the place.”
- The comment was made that we should “always be building in how we’re going to train people in AI use. I think this is a really important part that doesn’t always get included.”
- Communicating the power of AI to the general public and public officials will help to alleviate the fears of the unknown that almost all new technologies present. “It’s a matter of transparency,” said one participant, “and not just with your internal stakeholders.”
One final challenge about which panelists expressed concern was the use of image generators to classify individuals. One well-known image generator can be used to turn text into images, including realistic photos of human beings.
The risks are clear. As one article reported, “Some experts in generative AI predict that as much as 90% of content on the internet could be artificially generated within a few years. As these tools proliferate, the biases they reflect aren’t just further perpetuating stereotypes that threaten to stall progress toward greater equality in representation — they could also result in unfair treatment. Take policing, for example. Using biased text-to-image AI to create sketches of suspected offenders could lead to wrongful convictions.”
Well-recognized and commonly recommended methods for addressing biases in image generators include the following: ensuring data diversity and quality, implementing fairness-aware algorithms, incorporating human oversight, maintaining transparency and accountability, conducting regular audits and updates, raising public awareness and educating users, and developing and enforcing ethical guidelines and regulations.
The need to find solutions for the problems that will inevitably crop up as AI becomes ubiquitous in the public sector may seem to be an endless quest in a brave new world in which each new advance in the use of AI brings with it a whole new set of issues to confront. For now, however, there are a developing number of ways available to deal with this whole new set of issues, and many government leaders discussed these at the gathering.